In July, NYP AI made waves with our "Fun with AI 2023" workshop. What set it apart? Not only was it one of our largest gathering with 80+ participants, but it was also tailored for beginners. Even those without a coding background found themselves diving deep into the world of AI, discovering the technology that's reshaping our world.
Games, AI Ethics, and More!
Before diving into the hands-on session, the workshop set the mood right with some intriguing games. One such game required participants to distinguish between AI-generated and real images. It was not just fun but served a dual purpose to show the astonishing capabilities of AI in mimicking reality and how it should be used responsibly.
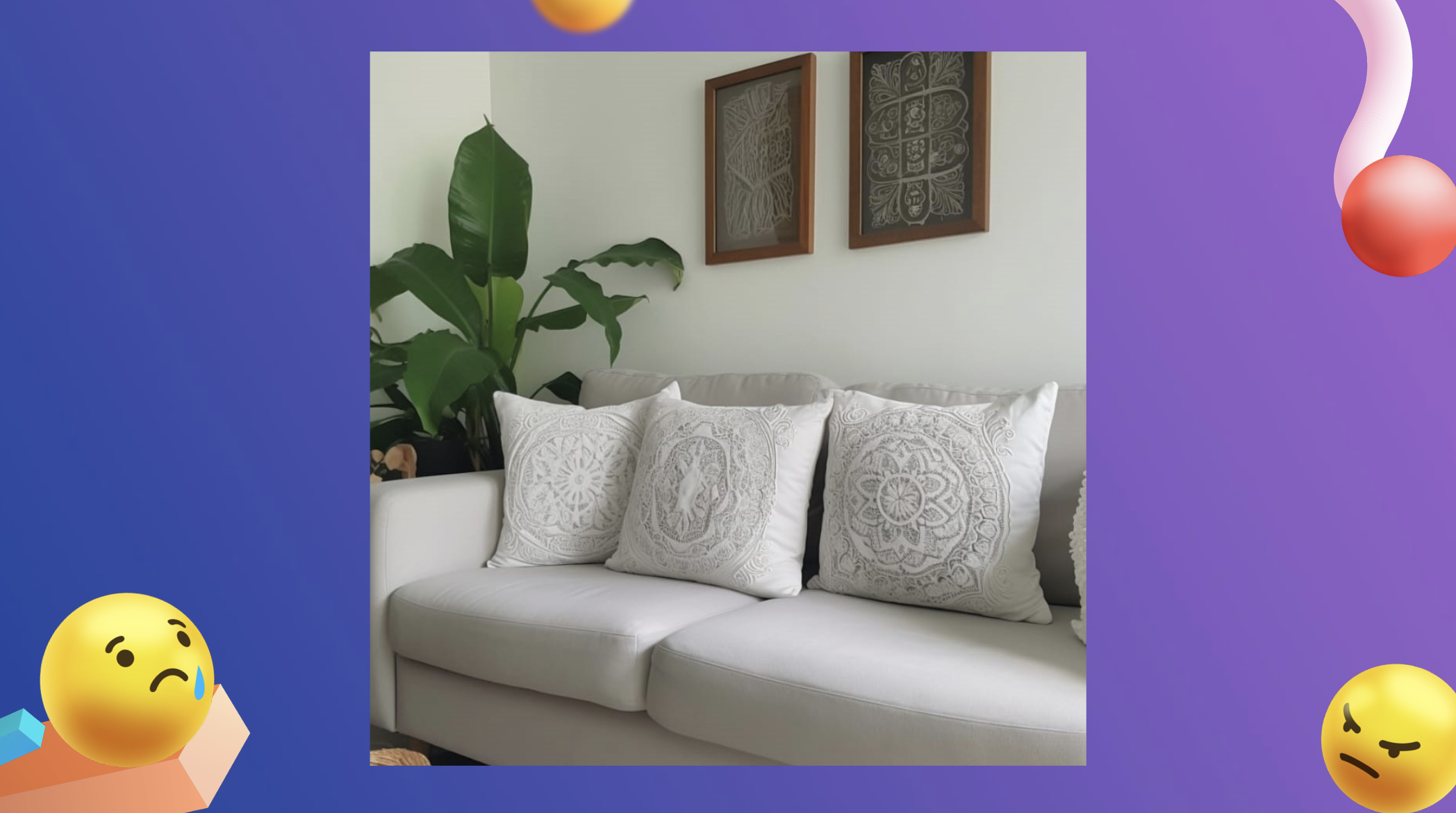
This game sparked insightful discussions about AIโs potential, its limitations, and the responsibilities of those harnessing its power.
Concepts Covered
Before we moved on to the hands on portion of the workshop, we covered some of the theory on some basic AI and computer vision concepts and how everything is related together.
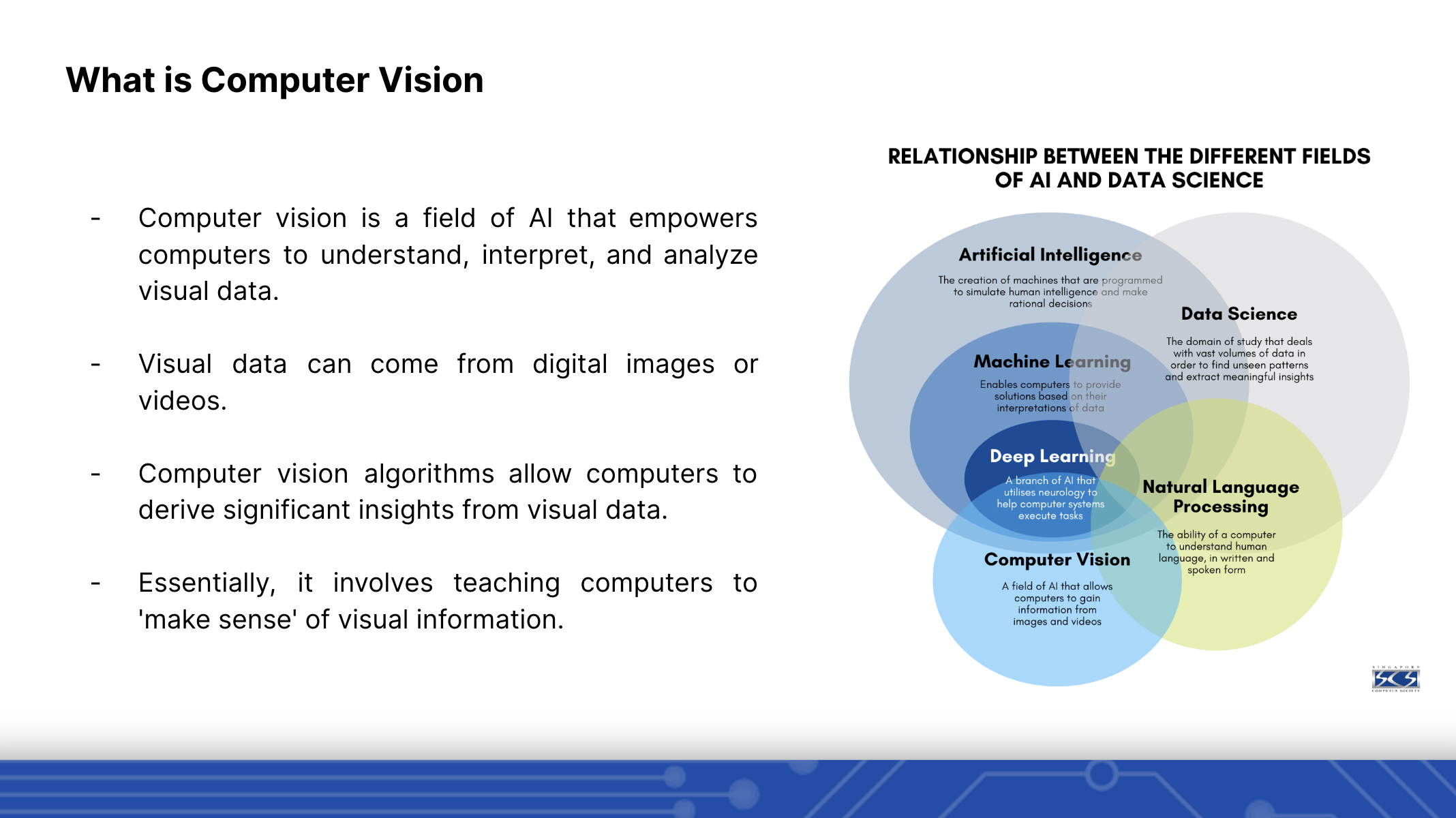
Since we were more focused on the computer vision part, we spoke more about some of the tasks that can be acomplished such as Image Classification, Object Detection, Optical Character Recognition (OCR), etc.
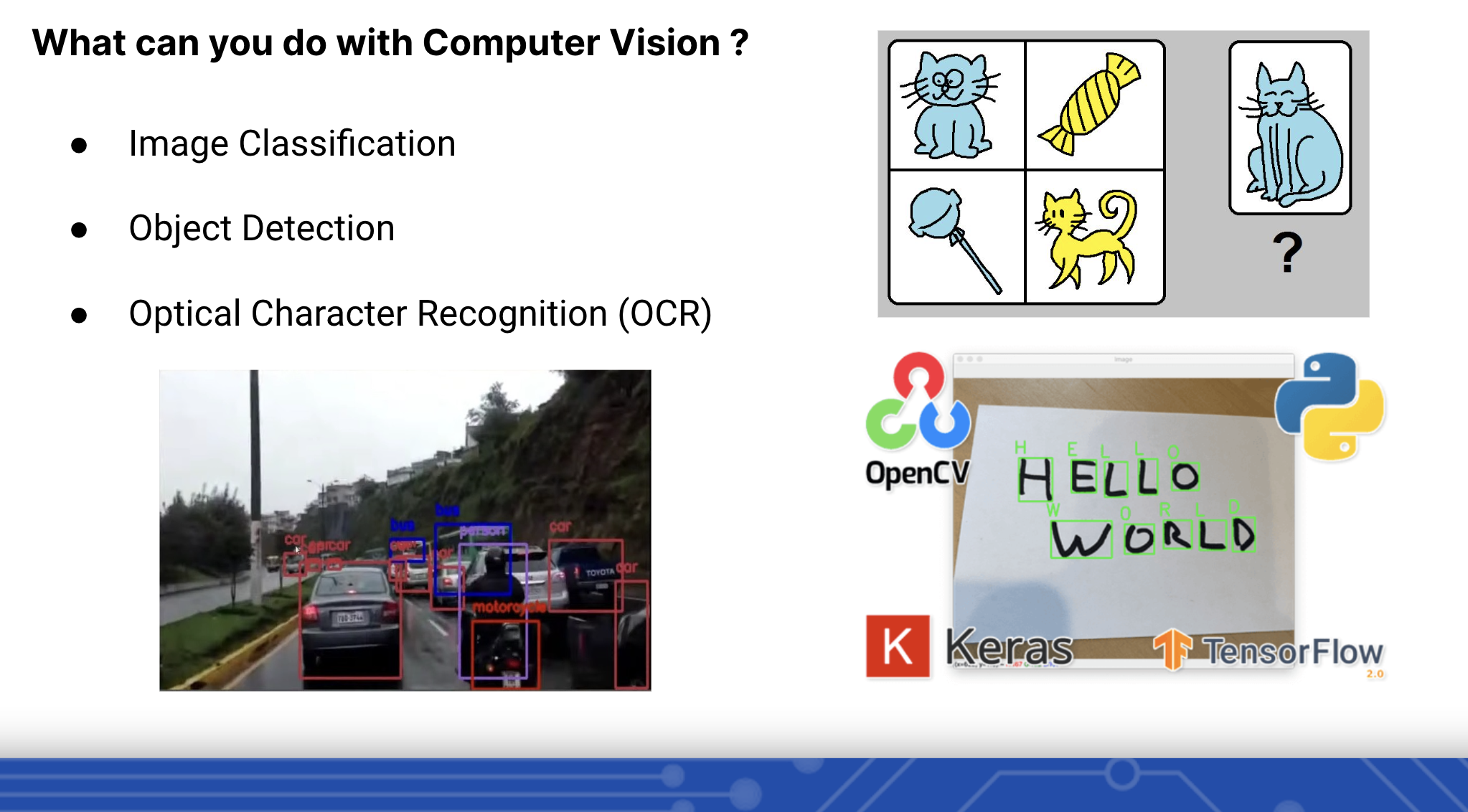
In any machine learning project, we start with data collection. This data can be messy, so we clean and organize it through preprocessing. For computer vision, preprocessing means adjusting image sizes, fixing brightness, and sometimes flipping or rotating images to get more variety.
Once the data is ready, we train our model. We use the cleaned-up images to teach the model how to recognise patterns and objects. The quality of the data we collect at the start plays a big role in how well our model will work.
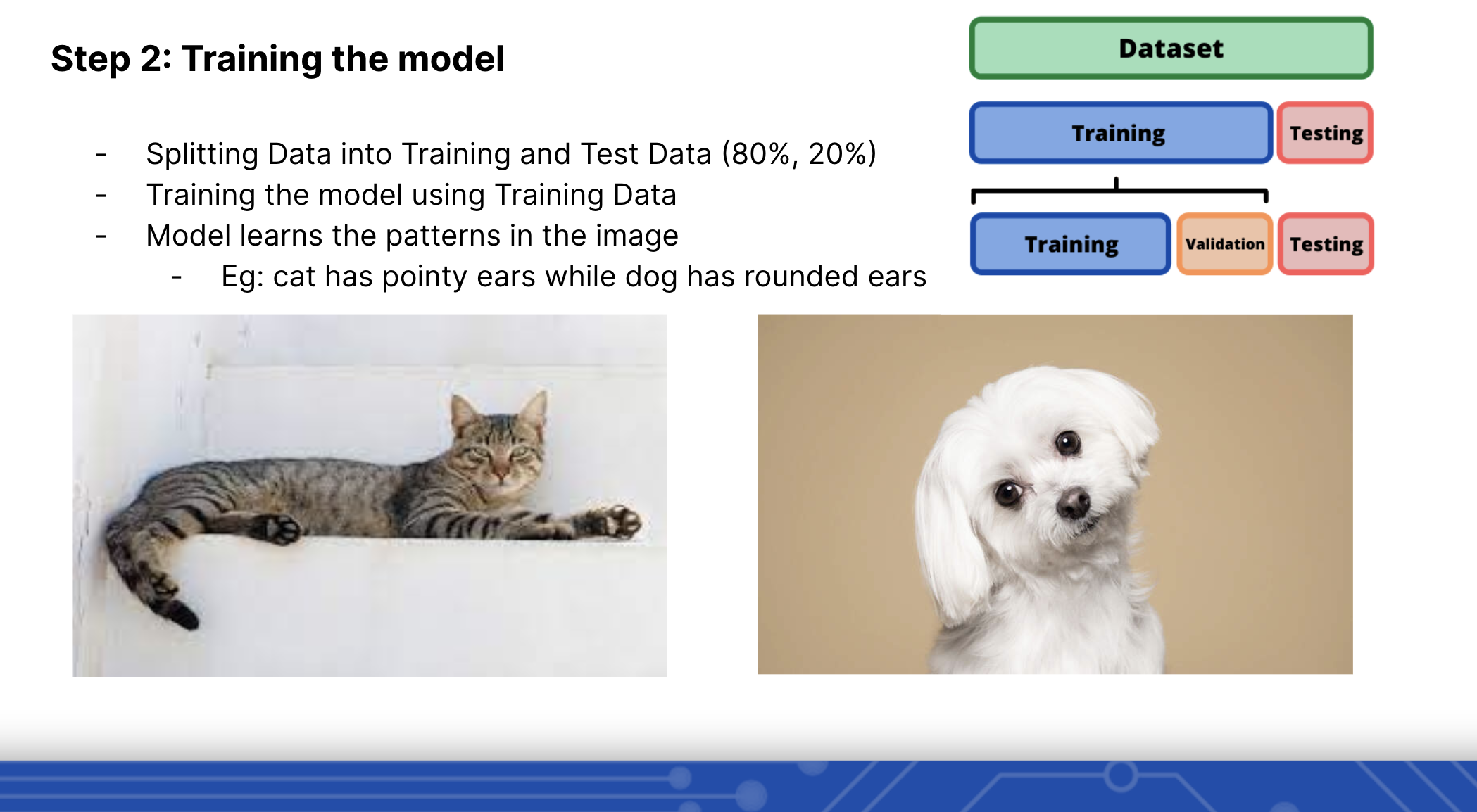
While our discussions often revolve around the intricacies of building models, this workshop placed a heightened emphasis on evaluation. After all, it's the quality of evaluation that truly determines the merit of a model, rather than the complexity of the modeling techniques used. The primary focus was on accuracy, but we also introduced participants to metrics like the ROC curve, true positives, and false positives. However, we kept our exploration of these metrics surface-level to avoid overwhelming the participants.
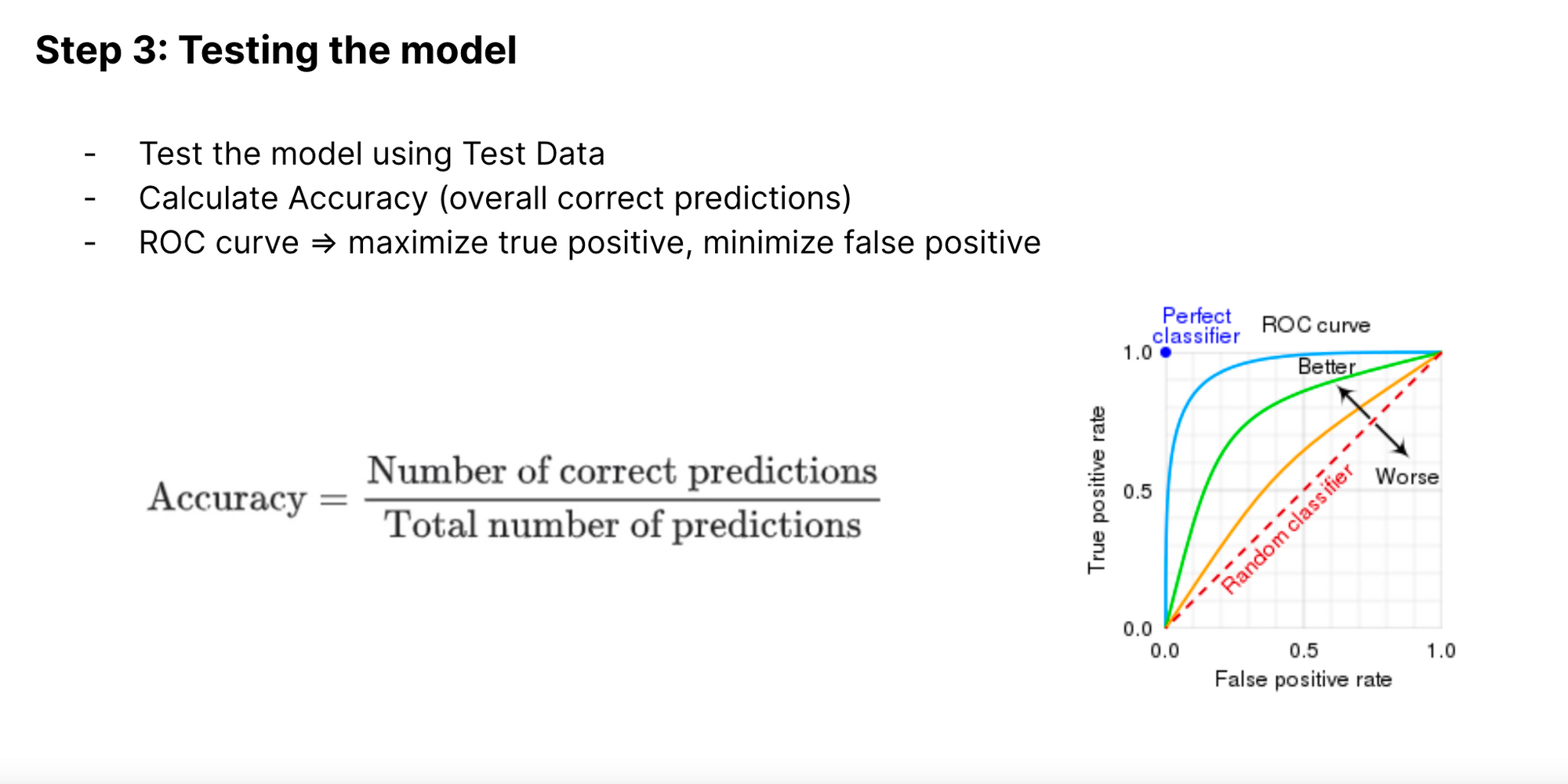
Following our discussion, we challenged participants to apply their newfound knowledge with a hands-on session using Teachable Machine.
No-Code AI with Google's Teachable Machine
Teachable machine is a tool that offers a no-code solution for machine learning, making AI accessible and understandable to all, regardless of their programming experience. For many participants, it was their first brush with AI, and the simplicity of Teachable Machine ensured they werenโt intimidated. With a user-friendly interface, participants easily created models, teaching their computers to recognise patterns, objects, and more.
While individual experimentation with the Teachable Machine was insightful, the event took a collaborative turn by introducing a group work component. This allowed participants to brainstorm, share ideas, and problem-solve collectively. It was not just about learning the tool, but about collaborative innovation, simulating real-world scenarios where teamwork often leads to. Some of the projects that the participants have worked on were on emotions identifier, among us classifier, dangerous tools detector, and more. After participants finished they then presented their models which allowed others to provide feedback and gain a deeper understanding of the topic.
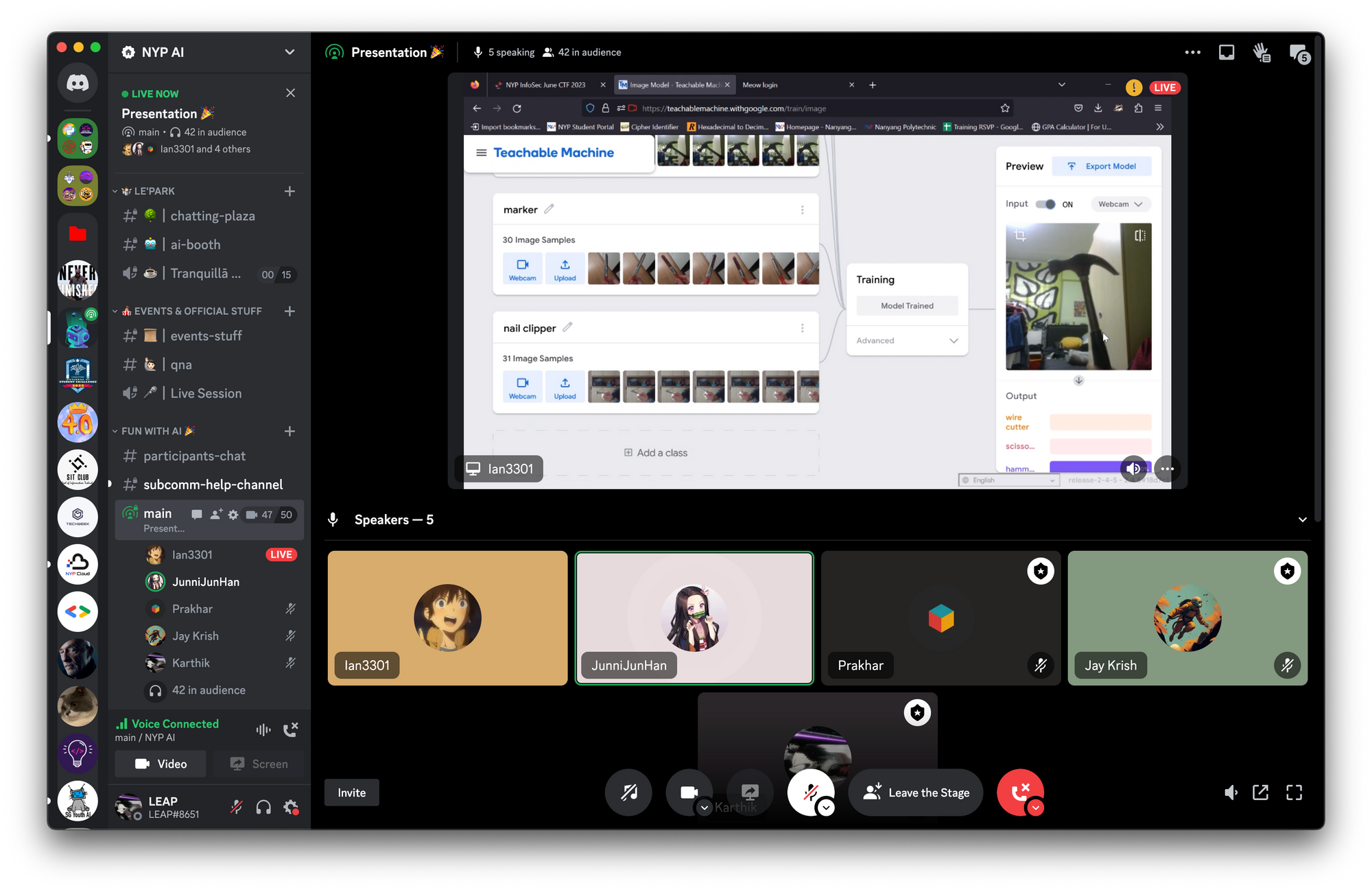
More of the models created by participants can be found at this link: https://padlet.com/prakhar0706/nyp-fun-with-ai-workshop-submissions-2b6xk1i37i53nl1e
All in all, the feedback that we received from this event was very positive largely due to the hands on portion of the workshop where participants were able to apply their own knowledge on use cases they are interested in. This shows that we should also consider including this for a more engaging event in the future.